Understanding Data Inequality
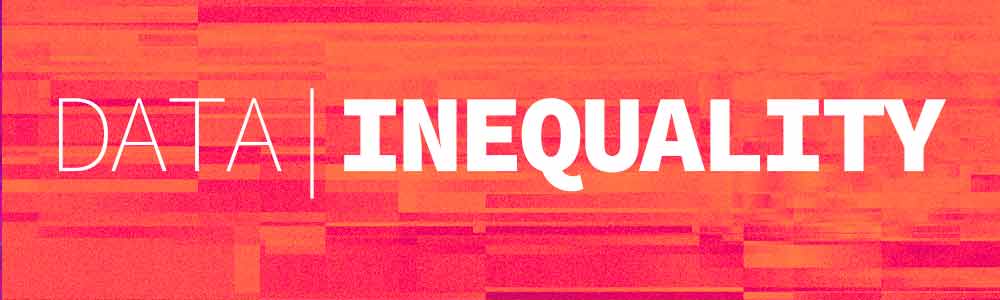
In this post, we explore how data inequality shows up in common business, communications, marketing, and technology decisions. We also offer several action items to address this growing problem.
Like its 2020 predecessor, the 2024 U.S. election was riddled with false narratives. Unfortunately, several significant challenges continue to plague the American democratic process:
- New tools such as generative AI make it easy to create convincing media and stories.
- People and organizations knowingly share or are otherwise incentivized to share false information.
- Lack of effective content moderation by social media platforms allows targeted disinformation to spread to millions of people.
In fact, in their Election Misinformation Tracker, watchdog organization NewsGuard reported:
- Nearly 1,000 websites regularly published false or misleading claims about elections.
- 793 social media accounts and video channels associated with publishers were flagged for repeatedly publishing false or egregiously misleading claims about elections.
- What’s more, 1,283 partisan sites masquerade as politically neutral news sources without identifying to readers where their funding comes from.
Predictably, racism, misogyny, transphobia, xenophobia, gaslighting, conspiracy theories, authoritarianism, and many other social problems thrive in this environment.
With that said, data inequality is not only about targeted disinformation. While misinformation and disinformation play key roles in many forms of data inequality, it is an increasingly complex topic that also includes accessibility, algorithmic bias, copyright issues, skills and wage divides, and other elements.
Data inequality also drives multiple business, communications, and marketing challenges as well. These challenges impact organizations across industries and sectors, causing widespread issues.
In light of this, let’s explore common threats to more equitable data ecosystems. Let’s also talk about ways to address these issues within our own organizations.
Defining Data Inequality
Inequality resides not only in having or not having data, but also in having or not having the power to decide what kind of data is being generated and in what form or format, how and where it is amassed and used, by whom, for what purpose, and for whose benefit.
— Angelina Fisher, Thomas Streinz, Confronting Data Inequality
Data inequality occurs from the control or use of data in ways that create power imbalances. It often stems from problems with access to data, data representation, or in data governance workflows.
Unsurprisingly, data inequality follows existing axes of exclusion along lines of:
- Class and social standing
- Race and ethnicity
- Gender and sexuality
- Education
- Age
- Physical and cognitive abilities
- Urban vs. rural geography
- And so on…
Data inequality also spans industries, geographies, and demographics, especially in areas like healthcare, employment, security, credit scoring, and, of course, marketing.
Many data inequality problems stem from a widely pervasive view of data as a ‘natural’ resource for extraction and exploitation. For example, Big Tech platforms and their disciples:
- Often spread the common narrative that technology is society’s great savior.
- Offer ‘free’ products and services, except you pay for these with personal information which comes at a significant cost to your privacy, mental health, and well-being.
Unfortunately, as technology in modern society is more ubiquitous, data inequality is more widespread, as are the many problems associated with it. Plus, rapidly expanding artificial intelligence exponentially multiplies data inequality issues.
Moreover, current regulatory guidance and industry ‘best practices’ barely scratch the surface of what is needed to make tech ecosystems more just and equitable. We all have a role to play in improving these systems. Here’s how.
Threats: How Manipulating Data Leads to Inequality
Suppose I have a theory that wearing red shorts causes shark attacks. To ‘prove’ this theory, I research shark attacks that happen where the victim wore red shorts. Every time I find an instance where that happens, I pat myself on the back and tell everyone how smart I am. However, such an approach ignores instances where someone wore red shorts and was not attacked by a shark. Such data would invalidate my theory; however, I never find them because I never look for them.
— Alan Klement, When Coffee and Kale Compete
The web is a statistics machine. Publishers and content creators share new research reports, infographics, and white papers on everything from climate change to sales enablement every day. In fact, the content marketing industry—which was worth $413 billion in 2022 and is projected to reach nearly $2 trillion by 2032—relies on statistics to drive success.
Unfortunately, the quote above illustrates how statistics are easily manipulated or overlooked. When manipulated data is presented as fact, misinformation and disinformation thrive. In the process, we exclude or otherwise marginalize data stakeholders:
- Lack of access to accurate, truthful information,
- Misrepresenting people or events,
- Governance processes (or, often, a lack thereof) don’t provide equitable outcomes.
Undermining Effective Communications
This inhibits our collective ability to deliver meaningful or otherwise useful information via the web. In fact, in Bob Moore’s book, Ecosystem-Led Growth, the author outlines several disruptive challenges currently facing tech-enabled organizations that drive business via the web:
- Inbound marketing: AI floods the web with middling content that compromises search engines’ ability to meaningfully answer people’s questions.
- Outbound sales: Similarly, market saturation and ‘inbox fatigue’, coupled with regulations like GDPR and advanced spam filtering diminish the effectiveness of outbound sales efforts. Plus, AI plays dual roles here too by both increasing output on the sales side while simultaneously filtering recipients’ inboxes.
- Ad targeting: Data privacy regulations, ad blockers, and tracker-blocking features built into Apple’s iPhone reduce the effectiveness of targeted ads, despite advertising costs remaining high. Also, algorithmic bias and AI hallucinations lead to misinterpreted data points that don’t accurately represent people’s needs or preferences, further undermining ad effectiveness.
What does this mean for data inequality? When coupled with a fundamental business need to drive exponential growth via late-stage capitalism, improvements in efficiency almost always lead to rebound effects through increased adoption. These effects include:
- Reduced economic opportunities
- A rise in social justice issues
- Increased environmental problems
Creating Guardrails to Reduce Data Inequality
Data lives at the heart of these problems. Plus, we externalize many of these problems by passing adverse impacts onto society—through supply chains or via social media, for example. Organizations may not realize (or care) how they contribute to data inequality problems that face us all.
With this in mind, how might we put guardrails in place to address data inequality issues? The first step is to clearly understand how these issues show up in our day-to-day practices.
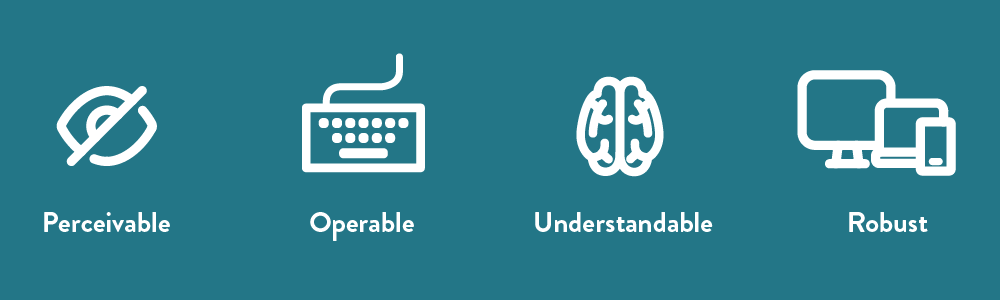
Three Forms of Data Inequality (with Examples)
Control over data conveys significant social, economic, and political power. Unequal control over data—a pervasive form of digital inequality—is a problem for economic development, human agency, and collective self-determination that needs to be addressed.
— Angelina Fisher, Thomas Streinz, Confronting Data Inequality
Below are three common forms of data inequality plus a high-level overview of who is impacted by these issues and examples of each. While this is not a comprehensive list, it should nonetheless provide an overview of how data inequality creeps into our everyday work.
1. Data Access and Availability
Information should be available and accessible to everyone, especially at times when it is needed most. This makes organizations more resilient and improves stakeholder relationships and sustainability while also reducing risk. However, reality often tells a different story.
For example, in their annual website accessibility study, disability rights advocate WebAIM found, among other things, that 96% of website homepages tested include accessibility errors. This undermines data access and availability, especially for people with disabilities who use assistive technology.
What’s more, lack of access to digital skills and tools—the ‘digital divide’—leaves underrepresented or otherwise marginalized communities unable to benefit from the outcomes of data-related practices.
Impacted stakeholders
- People with physical and cognitive disabilities
- People in low-bandwidth areas
- People who use older or slower devices or don’t have access to devices at all
- Non-English speakers
Examples
Ignoring Web Content Accessibility Guidelines plays a primary role. Reduced data access most often manifests through inaccessible checkout procedures, web forms, and other digital experiences that require user input.
However, other examples exist as well. For instance, companies sometimes don’t disclose energy use or emissions data. This makes meaningful Scope 3 emissions reporting next to impossible.
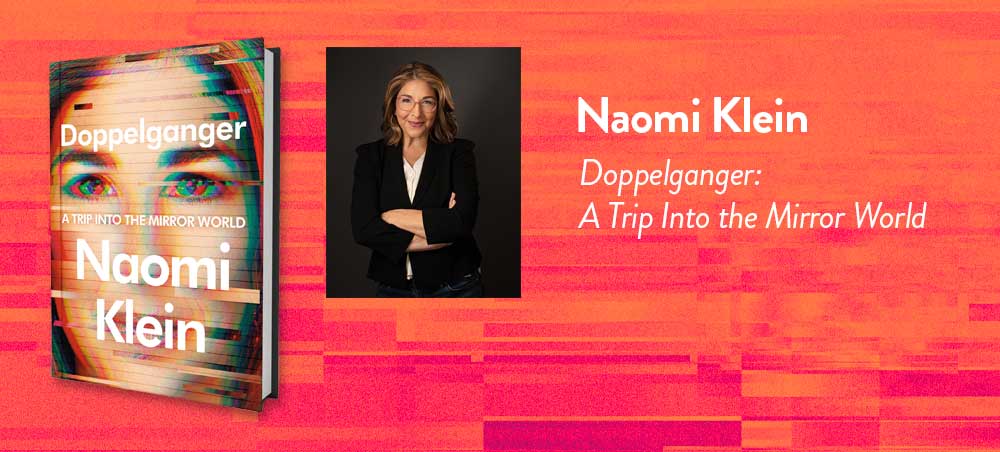
2. Data Representation
In the Mirror World, conspiracy theories detract attention from the billionaires who fund the networks of misinformation and away from the economic policies—deregulation, privatization, austerity—that have stratified wealth so cataclysmically in the neoliberal era. They rile up anger about the Davos elites, at Big Tech and Big Pharma—but the rage never seems to reach those targets. Instead it gets diverted into culture wars about anti-racist education, all-gender bathrooms, and Great Replacement panic directed at Black people, nonwhite immigrants, and Jews. Meanwhile, the billionaires who bankroll the whole charade are safe in the knowledge that the fury coursing through our culture isn’t coming for them.
— Naomi Klein, Doppelganger: A Trip Into the Mirror World
In Doppelganger: A Trip Into the Mirror World author Naomi Klein explores the complex world of data doppelgangers—what they are, why they exist, and how data representation inequalities undermine social structures. These inequalities arise when data is given an ontological power to represent the world based on diverse forms and sources of information.
However, this is always partial, its production always biased toward society’s most powerful—those who control systems through which data representation occurs.
In other words, as noted above, data representation drives marginalization. Because of this, data misrepresentation disproportionately impacts minority communities. Because of this, algorithmic bias leads to inequitable opportunities.
Impacted Stakeholders
- Social media: Anyone who uses online social media platforms is at risk.
- Web users: Even if you don’t use Facebook, Twitter, TikTok, or other social networking platforms, your data is still collected through millions of websites via cookies and related ad tracking scripts.
Unfortunately, these two groups alone represent billions of people around the world.
Examples
- Data doubles: Misrepresentation from faulty ‘data double’ categorization occurs, leading to Surveillance Capitalism mistakes.
- Crowdsourced data: Crowdsourcing information reinforces inequalities and often represents privileged perspectives, reproducing existing power structures.
- Algorithmic bias: We use generative AI to drive marketing strategy, create content, analyze performance, and improve measurement practices. However, biased or incomplete data in Large Language Models (LLMs), which form the basis of most generative AI tools, leads to inaccurate or unfair decision-making.
- Exclusionary language: Data presented using racist, ableist, gendered or other problematic language alienates people. So does data presented using overly technical language. This creates barriers to meaningful interactions and data access.
- Ideal customer profiles: Marketers use data-driven customer personas to help them identify demographics and character traits of audiences they hope to reach. Unfortunately, these surface-deep exercises often reinforce common biases, which causes misrepresentation that disproportionately impacts underrepresented or marginalized communities.
3. Data Workflows
[There is an] unequal accumulation of personal behavioral data and the inability to control how it flows between stakeholders – the people they represent (data subjects), the corporations that accumulate these data (data controllers), and governments and non-governmental organizations (data beneficiaries). This produces a divide between people and their data, and a divide between governments and other development stakeholders and the corporations that control these highly valuable sources of data for development.
— Jonathan Cinnamon, Data Inequalities and Why They Matter for Development
Good governance must drive more sustainable data strategies. Unfortunately, a lack of effective data workflow practices at many organizations creates gaps in how data are collected or managed. This leads to unintended consequences like customer frustration, increased costs, data breaches, potential lawsuits, and so on.
Big Tech’s business models require that we use more data, not less. This puts sometimes unnecessary pressure on organizations to prioritize data workflows or measure more than they need to. If an organization doesn’t have a clear strategy and data science champions within its ranks to execute this strategy, their approach to data quickly gets messy.
Impacted Stakeholders
- Crowdsourcing data platforms users
- Marketers, content creators, and agencies
- Gig economy workers (who often aren’t paid a living wage to fill organizational gaps)
- Data analysts and researchers
- Product and service teams
- Content moderators (who suffer from increased mental health issues)
Examples
- Data commodification: First, stakeholder data is not a commodity for extraction and exploitation, but rather a valuable tool that can help organizations strengthen relationships and create shared value. Organizations need to make this fundamental mindset shift.
- Terms of service: Egregious terms buried deep within lengthy legal documents plus a lack of transparency about data collection, privacy, etc. forces us to ‘accept or be excluded’ with limited ability to opt-out.
- Data privacy: Surveillance Capitalism separates people from their personal data, undermining privacy and potentially violating existing laws.
- Crowdsourcing: Data collected through crowdsourcing often includes participation and representation gaps which undermine data integrity and contribute to marginalization.
- Filter bubbles: Big Data platforms reduce people’s exposure to diverse perspectives and foster a polarized mob mentality that drives ‘us vs. them’ thinking. Plus, the pervasive ‘win-win’ narrative of Big Tech undermines our social fabric while spreading the message that tech will save us all.
- Digital skills divide: Who has access to tools and resources to create and manage data? Who doesn’t?
- Digital benefits/outcomes divide: Similarly, who receives the economic benefits of these efforts? Are these benefits shared in meaningful ways?
- Copyright: Intellectual property issues arise when AI companies don’t compensate creators when training their Large Language Models (LLMs).
- Third-party services: Finally, the modern web is built on products and services passing data between third-party providers that collect and distribute this data in ways that are inequitable and can lead to exploitation.
To address the litany of issues listed above, future legislation should give people autonomy over their personal information to support data privacy, fight disinformation, and improve data representation. To do this, however, many legislators must first be trained on how these issues affect people in their districts. See point #7 below.
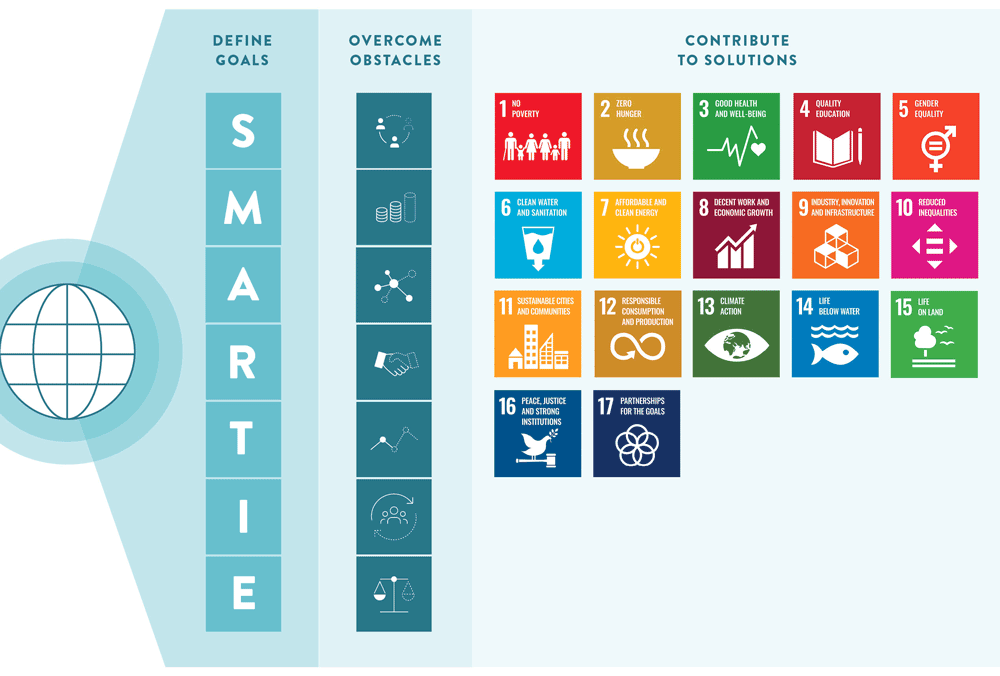
Action Items: Seven Ways to Improve Data Inequality
Decolonising data means dismantling extractivist models for collecting data, rejecting profit-driven views of our life as a territory ripe for dispossession, and turning data into a collectively owned tool for transforming the world in positive ways.
— Ulises A. Mejias and Nick Couldry, Data Grab: The New Colonialism of Big Tech and How to Fight Back
Here are seven intersectional practices to redefine success in your own communications and promote more equitable data access, collection, representation, and analysis across channels and disciplines.
1. Encourage Systems Thinking to Redefine Success
Exercises like stakeholder mapping can help teams shift their worldview. This helps organization drive systemic change and better understand networks, actors, and relationships that make up their business ecosystems.
The environment and third-party providers in your digital supply chain should be included as part of these exercises.
In turn, these collaborative processes should increase awareness of potential unintended consequences from data projects while also informing more equitable solutions. Consider trying these things:
- Collaborative workshops: Design thinking processes like the stakeholder mapping exercise mentioned above can help your team better incorporate systems thinking into organizational initiatives.
- Team training: If systems thinking isn’t a core team competency, invest the resources necessary to build skills and incorporate these practices into important activities, like annual budgeting, impact reporting, and strategic planning.
- SMARTIE goals: When defining strategic imperatives, use goals that are Specific, Measurable, Achievable, Relevant, Time-Bound, Inclusive, and Equitable.
2. Who Ensures Equitable Access and Representation?
Often, data sets used in AI development do not adequately represent people with disabilities, leading to AI systems that do not work well for this community. Inclusive data sets are crucial for creating accessible products that effectively serve people with disabilities.
— AI and Accessibility (video), Microsoft Guidelines for Responsible AI
Make sure it’s someone’s responsibility to prioritize data equality issues and track progress over time on your continuous improvement journey. It’s one thing to workshop a more equitable strategy or plan. It’s quite another to create enduring success over time. Someone needs to take ownership.
Whether this means appointing a single data leader or creating a cross-disciplinary data task force that meets regularly, responsible and more sustainable data strategies require an organization to build long-term capacity and good governance practices around this work. Make sure they’re asking, “Which stakeholders are not represented in our data ecosystem?”
Moreover, be sure to equip this person or team with the resources necessary for enduring success:
- Decision-making: Share appropriate decision-making power with stakeholders to co-create solutions and help people level up their skill sets.
- Data governance: Allocate organizational resources (time, budget, staff, consultants, etc.) to support better data governance over time among team members.
- Prioritize accessibility: Incorporate accessibility principles into data workflows. For example, present data using inclusive language. The Language, Please website provides style guidance on evolving social, cultural, and identity-related topics.
- Motivation profiles: Motivations are more important than behaviors and demographics, which can lead to marginalization when creating an ideal client profile. Focus your persona efforts on clearly understanding stakeholder motivations.
- Open data: Balance transparency and interoperability with data privacy by embracing open data principles.
3. Align Organizational Policies with CDR Principles
CDR is a framework; it is like glue, connecting data, AI, sustainability, privacy, accessibility, trust, cybersecurity, and many other areas into one. It is there to help people and organizations maximize the way in which they impact people, society, and the planet, to help grow and improve reputation, and to help consciously question and reduce the potential negative effects that could occur.
— IEEE, Corporate Digital Responsibility: Securing Our Digital Futures
Corporate Digital Responsibility (CDR) includes seven foundational principles geared toward improving economic, social, and environmental impacts associated with a company’s digital products, services, programs, and policies. Equitable data strategies live at CDR’s heart.
If your organization struggles to operationalize policies and practices related to data inequality, CDR is an impactful framework to consider. The IEEE white paper linked above offers clear processes to get started.
4. Create Awareness of Potential Data Inequality Harms
Next, because data inequality issues are so complex and often misunderstood, anything you can do to call attention to when they occur is useful. Better yet, if you have designed practices to successfully address data inequality issues in your own work, share those efforts so others can learn.
- Internal practices: Model more inclusive and equitable behavior in your own data governance policies. Help your team build knowledge and capacity in this area.
- External communications: Call attention to data inequality in campaigns and communications. Share challenges and wins by publicly tracking your progress over time.
5. Encourage Transparency and Accountability from Data Platforms
Few organizations manage their data without help from a multitude of third-party services. Encourage transparency from these suppliers by engaging them directly to improve their policies and practices.
- Outreach: First, ask about policies if you cannot find public-facing commitments beyond obligatory privacy statements.
- Public ask: If you don’t receive a response from emails or website queries, make a public ask via social media. If possible, reference and link to good examples in your post.
- Supplier surveys: Next, conduct supplier surveys to better understand partner commitments and efforts.
- Supply chain: Finally, fill your supply chain with better partners (see next point).
6. Find Values-Aligned Partners
An organization’s data suppliers are often key to creating enduring success through better business intelligence. However, despite repeated world-saving narratives, the tech sector is shockingly terrible at making—and sticking to—public impact commitments.
To find more sustainable partners that are better aligned with your values, look for accessibility, privacy, and sustainability statements or a clear Code of Ethics on potential partner websites. If that doesn’t work, find values-aligned partners with the B Corp search tool or resources like Charity Navigator or Great Nonprofits. Unfortunately, we haven’t found a comprehensive list of Public Benefit Corporations online. Does that exist? It should.
7. Advocate for More Responsible Tech and Data Policies
The global “free flow” of data through the internet relies on physical infrastructures and interoperability standards. The laws facilitating and protecting this “free flow” tend to ignore considerations such as where data accumulates, between whom it flows, and who ultimately benefits.
— Angelina Fisher, Thomas Streinz, Confronting Data Inequality
Finally, while internal practices and policies that support better digital governance within your own organization are important and necessary, we also need to improve standards and expand regulatory guidance to better cover tech-related data inequality issues.
However, given the topic’s complexity, devising a single regulation to address all the issues outlined above is incredibly challenging. Instead, we should address data inequality through a variety of regulatory guidance covering data privacy, AI, sustainability, accessibility, and so on.
To engage in responsible tech advocacy:
- Understand: Learn about various responsible tech issues through posts like this and others.
- Partner: Find organizations to partner with in your advocacy efforts.
- Educate: Meet with legislators to educate them on responsible tech issues.
- Introduce: Encourage lawmakers to introduce responsible tech legislation.
- Support: Build awareness of these bills through education and outreach.
- Enact: Finally, vote for regulatory guidelines to become law.
Data-Productive vs. Data-Extractive
…human supremacism constitutes the most ignorant prejudice of all, as it operates under the demonstrably false belief that the only measure of progress is that of Homo sapiens’ well-being. Subordinate to human ends, the living planet is reduced to a resource for the aggrandizement of the one chosen species.
— Christopher Ketcham, The Unbearable Anthropocentrism of Our World in Data
To prepare for this post, I reviewed numerous books, academic articles, papers, and posts on data inequality and related topics. In reviewing these (mostly) peer-reviewed sources, I learned that data inequality is a much deeper and more complex topic than I realized (hence the length of this post).
However, the extensive research process also helped me better understand how digital responsibility and web sustainability require systemic solutions that give equal consideration to economic, social, and environmental challenges associated with how we use and govern technology. We must center equitable data practices n this work.
Finally, unless clear legislation is enacted to address these issues, it is inevitable that new forms of social inequality will arise as new forms of data representation (and exploitation) take hold. We already see these problems in the election process needed to run a functioning democracy. If that’s not an urgent call-to-action, I don’t know what is.
As always, if you read through this lengthy tome and still have questions or thoughts to discuss, I encourage you to reach out via our contact form or message me on LinkedIn.
Responsible Tech Advocacy Toolkit
Advocate for responsible tech policies that support stakeholders with this resource from the U.S.-Canada B Corp Marketers Network.
Get the ToolkitHero and Klein image backgrounds altered from an original image on Freepik.